Everyone knows that artificial intelligence is becoming more common in healthcare. Most people know that AI now matches, and in some cases surpasses physician accuracy. Many people assume that combining human and AI into a sort of hybrid clinician results in the best performance. However, the story is more complicated.
There is a historical precedent for the superiority of human-machine hybrids. In 1997, chess grandmaster Gary Kasparaov was defeated by IBM's Deep Blue, the first time a world champion lost to a supercomputer. In subsequent years, the chess world shifted its focus from the question of whether a machine could defeat a human to the task of developing strategies for collaboration between human and machine to improve the level of play. "Centaur chess," a variation of the game in which human players and algorithms team up, rose in popularity. It soon became clear that centaurs were superior chess players. Indeed, most of the best chess matches of all time have been been played by human-computer hybrids.
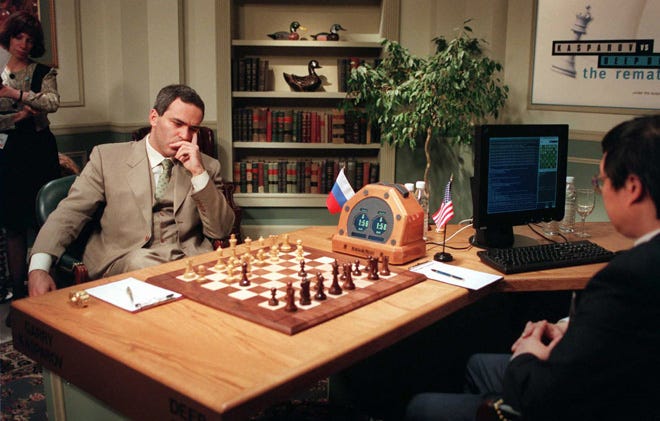
In medicine, it is often assumed that human+AI centaurs are more accurate than either on their own. A typical research study goes something like this: 1) code up a deep learning model to classify pathology on medical imaging, 2) demonstrate that the algorithm performs on par with human physicians, 3) show that the patterns of error made by human and AI are non-overlapping, and therefore 4) conclude that combining human and AI predictions results in best performance.
Perhaps unsurprisingly, it turns out the story is more nuanced in medicine. How do we optimally integrate AI into a clinician’s workflow to minimize harm and improve patient care? What is the most effective process for human-AI collaboration? Such questions are understudied, but the details of interfacing AI and humans in medicine are critical.

Early research on the impact of clinician-AI collaboration on patient care has been sobering. Marzyeh Ghassemi's group at MIT studied the effect of AI-based clinical decision support on physician performance, and found that accuracy markedly decreased when physicians received an incorrect prediction from the AI. For non-subspecialists (internists and emergency medicine physicians), their performance deteriorated to the point of being was worse than a coin flip. Andrew Ng's group at Stanford published similar findings in pathology; a deep-learning algorithm designed to assist pathologists in the diagnosis of liver cancer negatively impacted performance when the AI prediction was incorrect. These findings are consistent with a broader literature beyond medicine that demonstrates the potential for automation and decision-support aids to bias human decision making.
The history of centaur chess emphasizes the importance of process in effective human-machine collaboration. In 2005, a “freestyle” chess tournament was organized where teams comprised of both humans and computers could compete. Kasparov reflected on the surprising results of the tournament in his 2017 book Deep Thinking:
The surprise came at the conclusion of the event. The winner was revealed to be not a Grandmaster with a state-of-the-art PC, but a pair of amateur American players, Steven Cramton and Zackary Stephen, using three computers at the same time. Their skill at manipulating and “coaching” their computers to look very deeply into positions effectively counteracted the superior chess understanding of their Grandmaster opponents and the greater computational power of other participants. It was a triumph of process. A clever process beat superior knowledge and superior technology. It didn’t render knowledge and technology obsolete, of course, but it illustrated the power of efficiency and coordination to dramatically improve results. I represented my conclusion like this: weak human + machine + better process was superior to a strong computer alone and, more remarkably, superior to a strong human + machine + inferior process.
Cramton and Stephens were amateurs, but they were able to beat grandmasters and state-of-the-art algorithms by focusing on the process of integrating human and machine. For example, they maintained a database of each player’s strategies, and for which situations each strategy was best-suited. They systematically studied the strengths and weaknesses of the algorithm. “We had really good methodology for when to use the computer and when to use our human judgement, that elevated our advantage,” Crampton summarized in a BBC interview.
What is the optimal process for integrating AI and physician? Suchi Saria’s lab at Johns Hopkins, together with her startup Bayesian Health, published a trio of landmark papers last week on an AI early-warning system for sepsis that address this question. In retrospective and prospective data, Saria’s team demonstrated that the real-world deployment of an AI system improved the detection of sepsis at significant lead time (>5h earlier), reducing morbidity, mortality, and length of hospital stay. Equally impressive was the high rate of clinician engagement with the AI system - 89% of alerts were acknowledged and evaluated by the clinician. Adoption of sepsis alert systems has historically been a huge issue - most physicians mindlessly dismiss sepsis alerts most of the time (To better understand the cacophony of the hospital and the issue of “alarm fatigue,” check out the audio recording below from my ICU rotation in med school).
Most relevant to the present discussion was a third paper from Saria’s group that unpacked the variables that influenced clinician adoption of the AI system. A few themes were identified:
Clinicians perceived the AI as augmenting, and not replacing, their clinical judgement. As the title of paper highlights, “human-machine teaming” and trust was key to adoption.
Clinicians did not understand the details of the underlying algorithm. While they appreciated that the ML system was an improvement on traditional clinical-decision support, clinicians did not understand the difference in methods (i.e., traditional CDS is a rules-based algorithm that checked whether parameters exceeded a pre-defined threshold, whereas Bayesian’s system used an ML model trained and validated on a multi-dimensional dataset to generate a predictive risk score).
That said, clinicians that adopted the AI established trust with the system by developing a mental model of how the system worked. Clinicians were less concerned with the statistical details of the underlying algorithm, but rather with observing the system’s behavior in different clinical contexts. Like the amateur freestyle chess players, physicians developed an understanding for the limitations of the AI. The authors note:
Clinicians also differentiated their diagnostic process from the capabilities of ML, emphasizing elements of clinical expertise and intuition that they felt ML could not replicate. Multiple providers referenced the visible cues and richer information available from interacting with the patient at the bedside. An ED physician expressed, “[The system] can’t help you with what it can’t see.”
In summary, trust between physician and AI was a key factor in driving adoption. Trust was established through experience; like in chess, physicians developed mental models for the strength and weaknesses of the AI by observing the system in a range of clinical scenarios.
A knowledgeable reader may wonder about the role of explainable AI in fostering human-AI collaboration. It is fashionable in healthcare to emphasize the importance of interpretable AI - algorithms with an inner logic that can be understood by humans. Trust of the AI, the argument goes, is best established through transparency of the model and its predictions. On this point, I am increasingly unsure. First, it assumes that existing explainability techniques produce accurate human-comprehensible explanations, but this is increasingly doubtful. Second, AI explanations may actually impair human-AI performance in certain situations. When AI predictions are incorrect, providing human-comprehensible explanations alongside the prediction increases the likelihood that the incorrect predictions will be accepted, likely due to inappropriate trust of the algorithm. Further, there is scant evidence that augmenting AI predictions with explanations improves human-AI performance.
The history of AI in healthcare thus far has largely been a story of technical achievements demonstrating the feasibility of developing algorithms that rival human performance on a range of clinical tasks. Impressive, to be sure, but so far insufficient to impact patient care at scale. The path forward will require a shift in focus from human vs AI to human+AI, where the details of integrating and interfacing human and algorithm matter. The field will benefit from further study of questions like:
What is the right balance of trust vs skepticism in an AI system? How is this established?
How should UI/UX interfaces be designed and how should AI-derived predictions be delivered to avoid inducing cognitive biases in the user such as anchoring or confirmation bias? How should individual differences in users (e.g., expertise) be accounted for?
How should physician operators of AI systems be trained? What are the best strategies and processes for clinicians gaining experience with AI tools and gaining knowledge of strengths/weaknesses in a range of clinical contexts?
Curt Langlotz, a radiologist and Director of the Center for Artificial Intelligence in Medicine and Imaging at Stanford, famously said “AI won’t replace radiologists, but radiologists who use AI will replace radiologists who don’t”. I’d qualify this statement - AI won’t replace radiologists, but radiologists that effectively team with AI will replace radiologists that don’t .